What exactly is artificial intelligence (AI) and what business does it have in higher education? Simply put, AI is an attempt to emulate human knowledge by programming extensive rules into computers. Through machine learning and expert systems, machines can produce patterns within mass flows of data and pinpoint correlations that couldn’t possibly be immediately intuitive to humans.
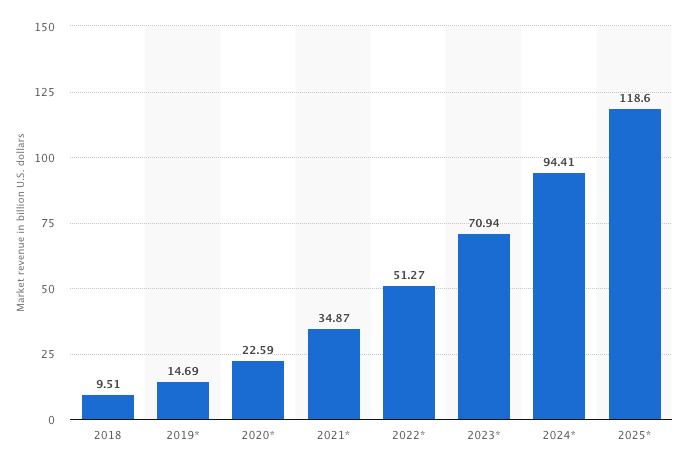
The developmental capabilities and precision of AI ultimately depend on the gathering of data – Big Data. Where better to find a continuous stream of information than within the highly active and engaging community of students. In saying so, the application of AI within higher education brings to light a mutually beneficial relationship between the two.
The future of artificial intelligence benefits from this interaction by gaining access to mass data upon which to draw inferences, identify correlations and build on predictive analysis strategies. In the same vein, artificial intelligence; built on machine learning, has been found to promote student success, provide opportunities for professional development and create personalized learning pathways.
At first instance, this appears to be a constructive advancement in both fields. As with most things in life, one should always consider the negative externalities produced by our conscious and collective decisions. The artificial exploitation of student’s digital footprints is no exception. Unsurprisingly, the application of artificial intelligence in higher education is not without its perils. But is it possible that the far-reaching benefits in doing so, outweigh any unwanted effects?
AI Applications Within Higher Education
“Colleges Mine Data on Their Applicants”, says the Wall Street Journal in an article on the way some universities are using AI and machine learning to determine prospective students’ level of interest in their institution. Individuals ‘demonstrated interest’ was inferred based on their interactions with university websites, social media posts and emails. Thousands of data points on each student are being used to assess admission applications.
The application of machine learning in the case above is a classic example of its institutional use. Other uses extend to student support, which for example, makes recommendations on courses and career paths based on how students with similar data profiles performed in the past. Traditionally this was a role of career service officers or guidance counsellors, the data-based recommendation service arguably provides better solutions for students.
Student support is further elevated by the use of predictive analytics and its potential to identify students who are at risk of failing or dropping out of university. Traditionally, institutions would rely on telltale signs of attendance or falling GPA to assess whether a student is at risk. AI systems allow for the analysis of more granular patterns of the student’s data profile. Real-time monitoring of the student’s risk allows for timely and effective action to be taken.
AI possesses the capacity to assist with individual students’ pace and progress. Some educational software systems analyses students’ data to assess their progress and make recommendations based on their skillset. Aimed at training business leaders and the future personnel of the digital world, University 20.35 introduces the first university model that uses AI to create individual educational trajectories by tracking digital skills profiles.
At Staffordshire University, they have a chatbot called Beacon – happily on hand to assist with any queries its students may have.
The collection of Big Data on a students’ educational and professional background, combined with his/her digital footprint allows the intelligent machine to suggest the best development path. Each student at any given moment can make a decision on their next educational steps based on recommendations that take into account their digital footprint, that of other students and the educational content available to them.
The resultant customized learning material makes for a solid foundation of effective learning and saves time that would be wasted on irrelevant material. Whilst the recommended educational trajectories are being crafted, so too, does the establishment of each student’s digital twin. In other words, the digital replica of physical assets (the student’s data profile) is representative of the student’s development on a real-time basis. The representation of an individual’s data, based on their digital footprint, as well as some biological data; can help to identify gaps in knowledge, forgetfulness and hone in on strengths.
Teamwork and group projects are important assessment strategies at university and train students to share diverse perspectives and hold one another accountable- a skill highly demanded in the workplace. The application of artificial intelligence in group projects at university is understated and underutilized. By configuring machine learning models to take into account each participant profiles, recommendations can be made to create the most efficient teams and hence produce the best results.
Perils of Depending on AI in Higher Education
As mentioned above – real-time monitoring of the student’s data profile to determine risk- may well be in the student’s best interest. But some data sets go as far as incorporating information on when a student stops going to the cafeteria for lunch or tracks their gym activity. Whilst this system helps to streamline success, it also raises important ethical concerns about student privacy and autonomy.
Having looked at some of the ways in which the process of using AI might be problematic, it should be noted that without due care and professional statisticians, adverse outcomes of relying on AI in the higher education system, might soon become apparent.
Models are based on correlation and are a great source of finding trends and patterns within datasets. However, they are not indicative of causation. Simply put, they offer us no answers as to why students digital skillsets shape up the way they do. On an extremely large scale, intelligent machines are pumping out correlations in virtually every direction. But distinguishing between the trends and patterns that are truly accurate, and those that are merely machine noise- can be difficult and still requires a lot of human input.
We take out what we put in. So, the quality and reliability of the data that goes into intelligent machines with be representative of the quality and reliability of the results it generates. Variations in quality or old and outdated data could produce unintended results and disrupt the entire application. There is also very little scope to monitor the quality of every student’s data.
The generalizability of the data could be detrimental to its applicability. Data that was drawn from a subset of the population or workforce, might not align with the students being targeted. Further, AI learning solutions that have been used on students in an ivy league school may not have the same outcomes and relevance for students in a community college elsewhere. The same spatial constraints are applied to notions of time. An AI system that was based on Millennial students may not be generalizable to digital learners of Generation Z.
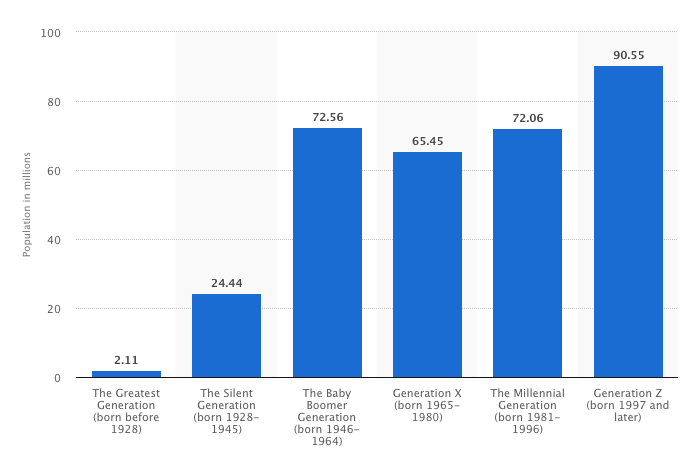
Promising or Perilous?
The bottom line is, artificial intelligence can be extremely useful in developing an advanced university experience. However, it’s not magic. Rather, computer systems that are created by humans and will for the foreseeable future, require human input.
A few key precautionary steps should be adhered to promote best-practice and to minimise risks of failure. First, intense professional training of those who will be implementing any AI strategy within an institution should prepare them to at the very least, be aware of the shortcomings.
Second, an effective oversight program within the institution should be in place to measure its effectiveness and plan for future developments of the artificial intelligence model in place. Another good way to create better results, in the long run, is to engage with students and faculty members to gain insight into their experiences and concerns with the technology.
Proceed with caution because, despite the potential risks associated with AI in the education system, the picture painted above is a beautiful one with a prosperous future.