Improving human health, longevity, and satisfaction are some of the primary purposes of technology. At first glance, artificial intelligence may feel like a step away from our humanity. But what if it could make us more observant, better able to find hidden connections, and more efficient as we explore the unknown?
Drug discovery and clinical trials are just one application area for AI, but it’s a deserving and impactful one. There are many medical conditions for which the “conventional” scientific method has not yet produced a treatment. Artificial intelligence can help us take better care of those we’ve left behind. In time, it will also help us balance the financial realities of the pharmaceutical industry with the moral imperative to render aid where needed.
Current R&D Models Provide Diminishing Returns
Clinical trials and R&D in the pharmaceutical industry have reached what some call a crisis point. To understand why, we must first understand the process.
Researching and developing new drugs involves multiple steps called “Phases.” Phase 0 is the first to involve human testing. It’s where scientists try to learn more about how the human body processes the drug. Phase I involves dialing-in the proper dosage and further testing in a larger patient pool.
Scientists learn more about the safety and effectiveness of new drugs in Phases II and III. This is also where new formulations receive apples-to-apples comparisons with existing “standard of care” drugs and methods. Unfortunately, a substantial number of clinical trials fails in these two Phases.
In a report on the failure rates of drug discovery efforts between 2013 and 2015, Richard K. Harrison (Chief Scientific Officer at Clarivate Analytics) found that:
- Almost half of new Phase II drugs are rejected because they do not demonstrate effectiveness against the targeted condition.
- More than half of Phase III drugs are rejected because they fail to demonstrate the desired effectiveness.
- Of all clinical trial failures between 2013 and 2015, 24% of new drugs were rejected due to safety concerns and 15% were rejected due to an incompatible corporate strategy (not profitable enough, the company was acquired mid-trial or went bankrupt, etc.).
The therapeutic areas hit the hardest by these failures are oncology, the central nervous system, and musculoskeletal disorders. Without better methodology, difficult-to-treat and ill-understood conditions and diseases are at risk of staying that way.
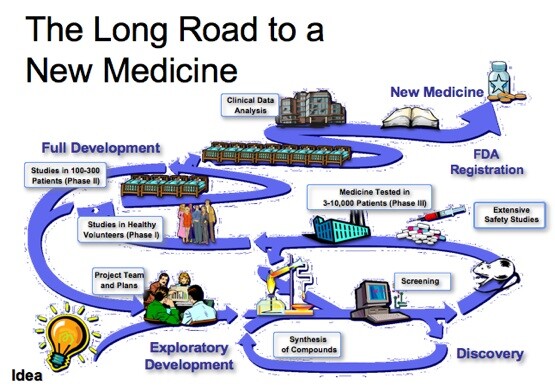
Existing Methods Leave Many Patient Needs Unmet
The greatest advantage of AI is that it can digest vast amounts of medical knowledge — from thousands of published reports and scientific papers, say — and devise novel predictions and formulations that would take human researchers years of inefficient experimentation to find.
It takes a decade, on average, for a drug to traverse the entire R&D process and achieve FDA approval. Instead, artificial intelligence gives us the means to uncover hidden links between an array of biomedical entities, such as proteins. Picture putting together a 100,000-piece jigsaw puzzle with the usual methods. Some of the pieces are missing. Now, picture the same process using heuristic models, machine vision, and artificial intelligence. Such an system would even be able to hazard a clinically useful guess about what the missing “pieces” look like.
The 15% failure rate of new drugs due to incompatible company strategies doesn’t have to continue. It’s understandable, though not exactly comforting, that pharmaceutical companies don’t want to sink their profits into drugs destined to cure only a small percentage of the population. Particularly not when there are expensive treatments available but not an actual cure.
According to Bloomberg News, pharmaceutical companies are even abandoning antiobiotic research at an unprecedented rate because there’s not enough money in it. As a result, governments are looking into alternative incentives to bring them back into the process. With mature AI systems to do the work at low cost, these incentives might not be necessary.
There’s also the problem of pharmaceutical companies overstating the effectiveness or omitting critical information from the clinical trial process. An open and impartial AI model should be able to inject a measure of transparency into this process along with the obvious efficiency advantages.
AI Casts a Wider Net for Clinical Trial Participants
Drug discovery efforts in the United States have long suffered under two unfortunate realities: Pharmaceutical companies often fail to recruit (1) A sufficient number of participants to see the program off the ground and (2) A patient sampling that accurately reflects the diversity of the population. Altogether, around one-quarter of cancer drug trials never even begin because there aren’t enough suitable participants.
Artificial intelligence can help. One way is to aid in the early detection of disease. Some AI systems render diagnoses with nearly the same accuracy as human doctors. This means patients in earlier or harder-to-detect phases of a disease can be discovered more quickly and in greater numbers, potentially boosting applications for new drug trials and providing a panel of patients at diverse stages of the disease.
AI can scour medical journals for unexplored connections between research projects and create never-before-seen drug formulas. With the right privacy safeguards in place, researchers can also use AI to find qualified drug trial candidates from much larger pools of patients.
Patient histories are long and complex. It’s often a subtle detail that renders an individual either an ideal or a poor candidate. Identifying patients who may respond well or poorly to an emerging drug is one of the most expensive steps in the entire progress.
To bring costs down and encourage further experimentation, artificial intelligence can study hundreds or thousands of patient records in search of the biomarkers the drug intends to target. The right kind of AI should be able to eliminate deliberate and unconscious bias from the selection process, too. It should ensure that drug discovery projects are truly representative of the public they’re intended to serve.
Artificial Intelligence and Medicine’s Bright Future
Is artificial intelligence a “silver bullet” here? Conventional methods for discovering new drug treatments involve lots of trial and error on the part of physicians and researchers. It’s a scavenger hunt through unknown chemical combinations.
The difference is, AI is orders of magnitude more efficient at hunting through the endless combinations and studying them in realistic digital models. It’s just what the medical community needs at a time when public health is a top-tier concern and when the costs for R&D seem to increase every year.