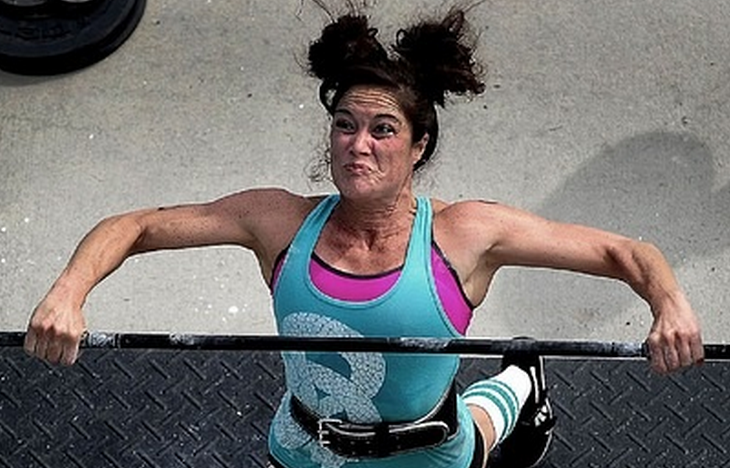
For a long time I’ve been a big proponent of weighting data as little and as rarely as possible. You see, I would rather fill cells to achieve the desired sample size rather than fake cells based on data I’ve already gathered – not on data that I failed to gather.
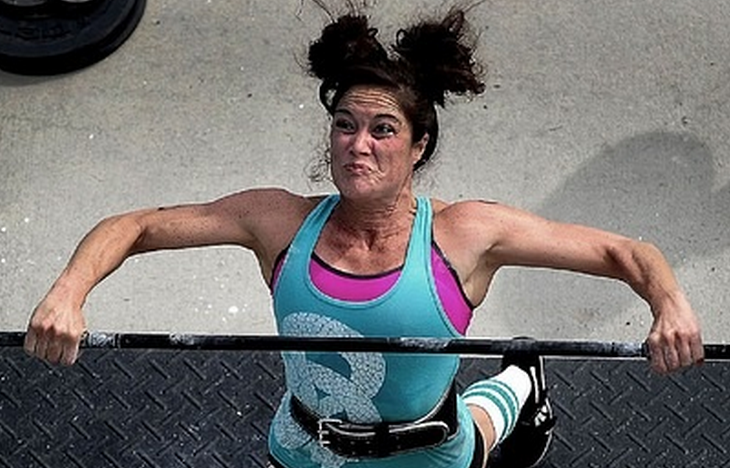
For a long time I’ve been a big proponent of weighting data as little and as rarely as possible. You see, I would rather fill cells to achieve the desired sample size rather than fake cells based on data I’ve already gathered – not on data that I failed to gather. Obviously, I failed to gather that data for a specific reason, perhaps one that is completely unknown and unguessable to me.
Let’s look at an example. I asked Excel to randomly generate a score from 1 to 10 for fifty men and fifty women. Then, I copied those scores four times. In the first copy, I deleted the last ten scores of women. In the second copy, I deleted the last twenty scores of women, and so on. What I was left with was five datasets based on the exact same population, but with various percentages of missing data. Why was the data missing? I don’t know. Maybe the introverts didn’t answer the door/pick up the phone/talk to me at the mall. Maybe the high income people were all away from home and at their cottages that weekend. Maybe my interviewer drove up in a beat-up, rusty Pinto and scared people off. Regardless, data analysis must begin!
In the Perfect Sample, we see the results from 50 men and 50 women wherein the weights for both men and women are 1, and both the unweighted and weighted total scores are 5.5. But notice what happens when data from more and more women become missing, and the weights for women must compensate and become higher and higher. Although we know that the “true” score is 5.5, the Unweighted Total Score eventually becomes 4.8 and is made even worse with the Weighted Total Score which eventually becomes 4.5 in Scenario 4. In other words, although weighting the data ensured that women’s opinions were counted just as much as men’s opinions, it didn’t improve the quality of the data. It simply exaggerated a flawed sampling technique.
Random Sample
Scenario 2
Scenario 3
Scenario 3
Scenario 4
Unweighted Total Score
5.5
5.3
5.1
4.9
4.8
Number of men
50
50
50
50
50
Number of women
50
45
40
35
30
Weight for men
1.0
1.0
1.0
1.0
1.0
Weight for women
1.0
1.11
1.25
1.43
1.67
Weighted Total Score
5.5
5.3
5.0
4.8
4.5
The moral of the story is this.
Never use weighting to adjust or correct for missing data.
Fill every cell, and then use weighting sparingly to adjust for extremely minor differences that resulted from oversampling (not undersampling).
This message has been brought to you by the letter α and the number 27644437.
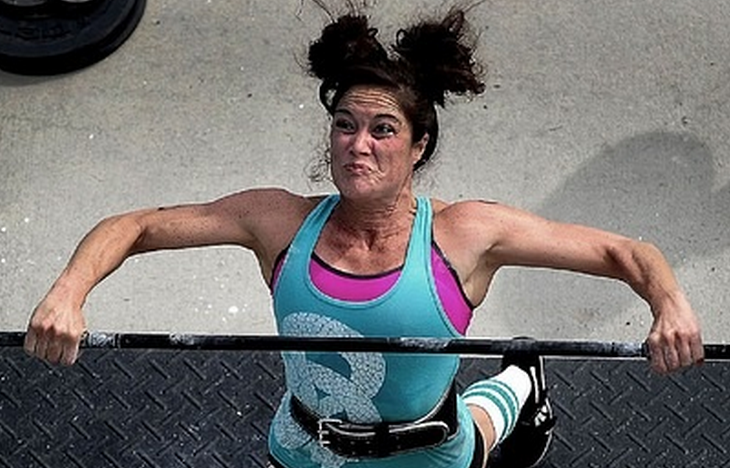
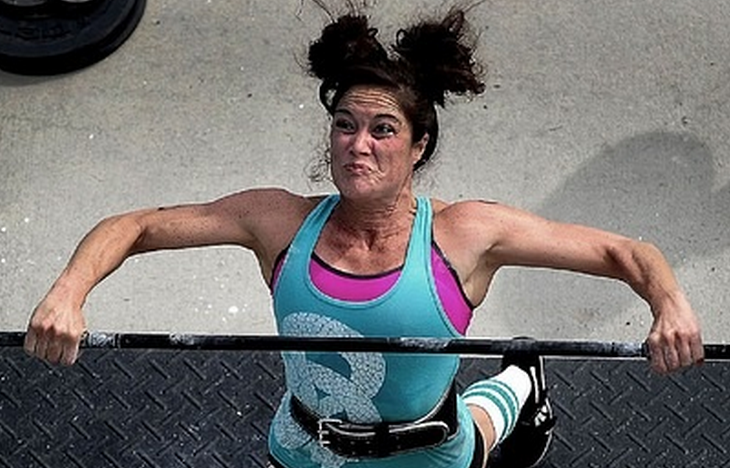
For a long time I’ve been a big proponent of weighting data as little and as rarely as possible. You see, I would rather fill cells to achieve the desired sample size rather than fake cells based on data I’ve already gathered – not on data that I failed to gather. Obviously, I failed to gather that data for a specific reason, perhaps one that is completely unknown and unguessable to me.
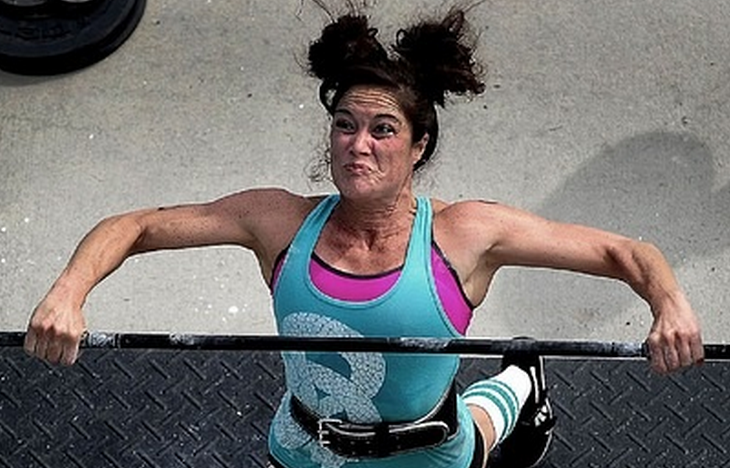
Let’s look at an example. I asked Excel to randomly generate a score from 1 to 10 for fifty men and fifty women. Then, I copied those scores four times. In the first copy, I deleted the last ten scores of women. In the second copy, I deleted the last twenty scores of women, and so on. What I was left with was five datasets based on the exact same population, but with various percentages of missing data. Why was the data missing? I don’t know. Maybe the introverts didn’t answer the door/pick up the phone/talk to me at the mall. Maybe the high income people were all away from home and at their cottages that weekend. Maybe my interviewer drove up in a beat-up, rusty Pinto and scared people off. Regardless, data analysis must begin!
In the Perfect Sample, we see the results from 50 men and 50 women wherein the weights for both men and women are 1, and both the unweighted and weighted total scores are 5.5. But notice what happens when data from more and more women become missing, and the weights for women must compensate and become higher and higher. Although we know that the “true” score is 5.5, the Unweighted Total Score eventually becomes 4.8 and is made even worse with the Weighted Total Score which eventually becomes 4.5 in Scenario 4. In other words, although weighting the data ensured that women’s opinions were counted just as much as men’s opinions, it didn’t improve the quality of the data. It simply exaggerated a flawed sampling technique.
Random Sample | Scenario 2 | Scenario 3 | Scenario 3 | Scenario 4 | |
Unweighted Total Score | 5.5 | 5.3 | 5.1 | 4.9 | 4.8 |
Number of men | 50 | 50 | 50 | 50 | 50 |
Number of women | 50 | 45 | 40 | 35 | 30 |
Weight for men | 1.0 | 1.0 | 1.0 | 1.0 | 1.0 |
Weight for women | 1.0 | 1.11 | 1.25 | 1.43 | 1.67 |
Weighted Total Score | 5.5 | 5.3 | 5.0 | 4.8 | 4.5 |
The moral of the story is this.
Never use weighting to adjust or correct for missing data.
Fill every cell, and then use weighting sparingly to adjust for extremely minor differences that resulted from oversampling (not undersampling).
This message has been brought to you by the letter α and the number 27644437.